As we talk about sustainability in food production, we consider new technologies in the first place. By applying smart strategies, we implement find smarter solutions than traditional farming offers. The drawbacks of traditional agriculture are the excessive usage of resources and fertilizers. They also don’t consider the increased pollution and soil degradation anymore. Besides, the traditional ways don’t meet the needs of growing food demands.
That’s why precision farming emerges as a new and promising solution. The union of advanced technologies and data analysis create a different approach to agriculture management. We have finally stepped into a path of sustainability in agriculture.
The Role of New Technologies in Precision Farming
The idea of precision farming is to collect big amounts of data through sensors, drone and satellite images, and other means. Once optimized for training AI models, they shape further decision-making on ways of managing farming.
What are the key players?
- Sensors. Sensors help to collect visual data through different modes and in different conditions. Installed on drones or satellites, they show the actual situation of crops. Whether in the form of images or videos, they generate the row data for processing and analysis. This is the basis for further agriculture data annotation services and machine learning algorithms training.
- Internet of Things (IoT). It allows transferring the data to the central network. As a result, we can organize a continuous monitoring of crops and store the data on the server.
- Artificial intelligence and machine learning. They are the basis for further model creation. After collecting and completing data annotation in agriculture, we move to the AI model creation. The machine learning algorithms are what actually allows models to predict and analyze.
- GPS. This navigation system is critical for field mapping and spatial analysis. It allows situating drones where needed for better performance optimization.
AI Tasks in Precision Farming
While AI continues to evolve, some settled strategies have already shown their efficiencies. In comparison to natural language processing that works with texts and audio, AI in agriculture is mostly based on images. AI models usually help with the following tasks:
- Crop monitoring. The general monitoring in real time allows detecting any anomalies in crops health. The monitoring provides data on patterns, color, and weed presence.
- Disease detection. Well-trained models identify the early signs of diseases from the crops images. However, the accurate results are only possible after a thorough data labeling in agriculture and successfully accomplished model training.
- Irrigation management. The sensors collect data not only on plants’ health, but also on weather and soil conditions. The algorithms prevent the need for irrigation and even suggest the duration and frequency of irrigation needed.
- Yield forecasting. The forecasting of yields takes place based on the actual and historical data on the crops. As a result, it helps to set strategies for further harvesting and planting seasons.
- Soil health management. As in cases of irrigation, the sensors in the soil provide valuable data on soil composition and health. The algorithms can recommend further strategies regarding the usage of fertilizers.
Data Annotation in Agriculture for Successful AI Model Performance
All the applications of AI in agriculture will be efficient if the model creation and testing are successful. To get the highest level of accuracy of a model prediction, it is important to thoroughly prepare the data for the AI model’s usage. Data labeling for agriculture helps with it, structuring and labeling all the raw data that is further used in AI training.
Data annotation for agriculture includes the following services:
- Semantic segmentation. This type of image markup allows associating objects on the image to different object classes. As a preparation stage for computer vision model training, it helps in a model’s accuracy of crop monitoring.
- Bounding boxes. Marking the objects on the image with frames, data labeling helps the AI models with pest localization and disease detection.
- Image categorization. With image categorizations, machine learning algorithms accurately differentiate different classes and groups of objects. This is especially beneficial for models monitoring livestock.
- Polygon annotation. Polygons help to classify objects, but they also work with shapes and boundaries. In agriculture, polygons are applicable for AI models analyzing maps and fields for crops planning or resource allocation.
The Importance of Data Annotation in Precision Farming
Precision farming will be successful only when the AI models are correctly built and trained. Taking into consideration the amount of raw data that comes in different formats, data labeling plays the role of a gatekeeper. It structures, labels, and classifies it accordingly. In the long run, annotation helps models to train quicker and efficiently perform their tasks.
Take, as an example, the detection of potential disease in crops. If a model doesn’t receive all the examples of potential diseases and how exactly the crops should look like, it will not recognize it. With data annotation, we provide to the models all the details to successfully pass the training and be able to identify the diseases later on.
The same applies for crop and soil monitoring, yield prediction, and research facilitation. Annotation helps AI models to tailor to farming needs. It increases the models’ accuracy and reduces the biased information. As a result, the analysis becomes more precise, enhancing resource optimization and improving crop management.
Managing Agriculture with Precision and Intelligence
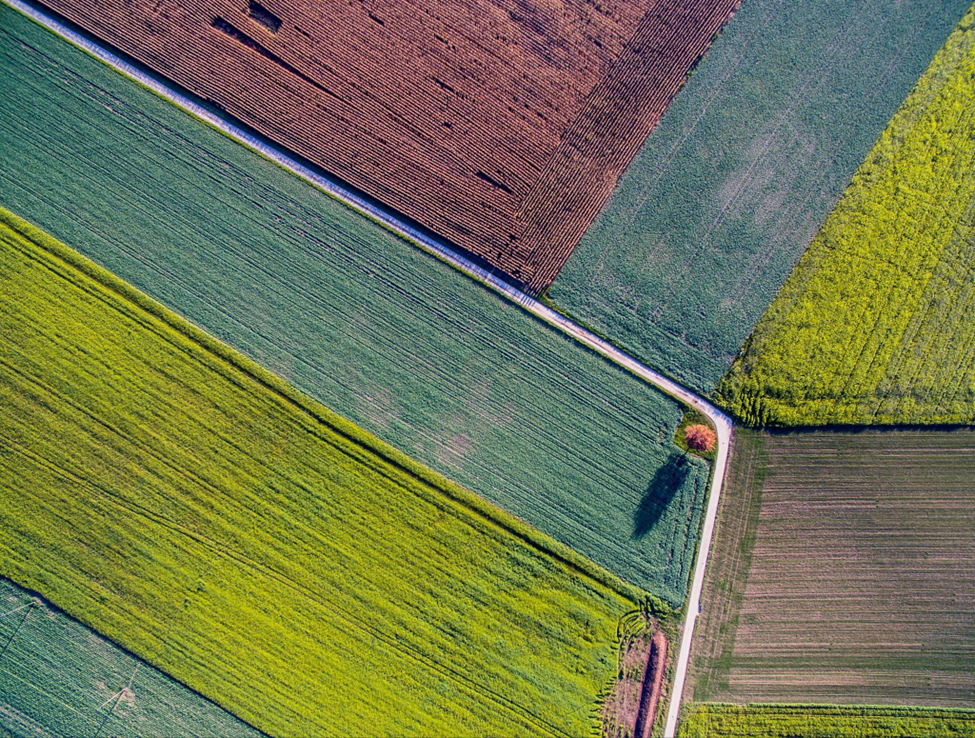
Photo by jean wimmerlin on Unsplash
Data annotation services are crucial in contributing to precision farming. They help to drive the agricultural industry towards greater efficiency, productivity, and sustainability. The meticulous data labeling in agriculture provides the foundation for AI models. As a result, they learn quicker, recognize patterns better, and make intelligent recommendations. This, in turn, empowers farmers to optimize resource use. Their strategies help to minimize waste, and make data-driven decisions that improve crop health and yields.
The usage of data annotation in precision farming helps with addressing the demands of growing global population. It also helps with responding to the increasing demand for food production. But it also paves the way for innovative and sustainable agricultural practices. With so many sophisticated tools in our arsenal and correct data management, we may hope to achieve even better results in our transition towards sustainable agriculture.